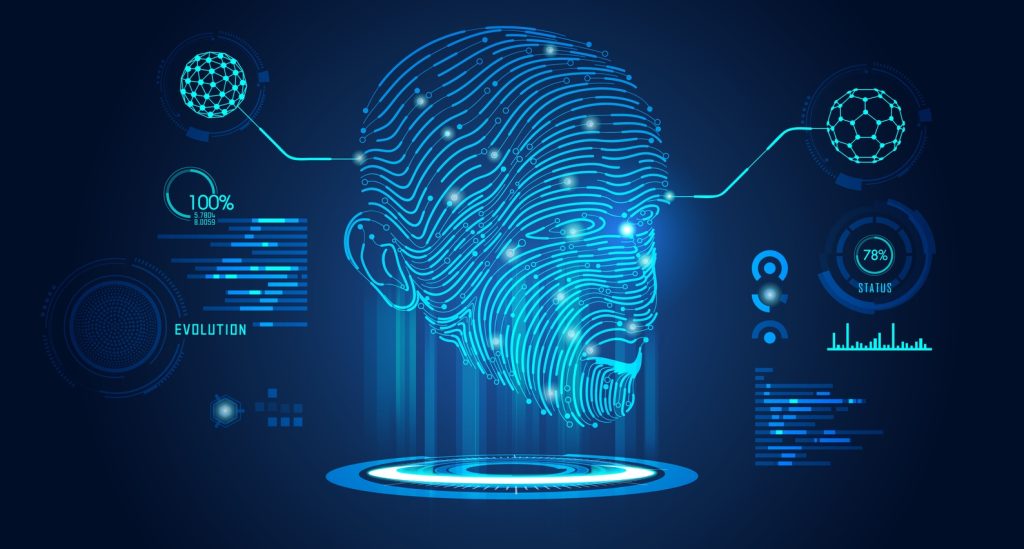
Artificial Intelligence (AI) and Machine Learning (ML) have made significant advancements in recent years, revolutionizing various industries and transforming the way we live and work. From self-driving cars to voice assistants, AI and ML technologies have become an integral part of our daily lives. In this article, we will explore some of the latest breakthroughs in AI and ML, highlighting their potential impact on society and the exciting possibilities they offer.
Deep Learning and Neural Networks
One of the most remarkable advancements in AI and ML is the development of deep learning algorithms and neural networks. Deep learning mimics the way the human brain works by creating artificial neural networks that can learn and make decisions based on vast amounts of data. This technology has led to significant improvements in image and speech recognition, natural language processing, and even drug discovery.
Deep learning has been particularly successful in the field of computer vision. Convolutional Neural Networks (CNNs) have demonstrated remarkable accuracy in tasks such as object detection, image classification, and facial recognition. For example, CNNs have been used to develop systems that can accurately identify diseases like skin cancer from images, potentially assisting doctors in early diagnosis and treatment.
In natural language processing, deep learning has enabled breakthroughs in machine translation, sentiment analysis, and text generation. Recurrent Neural Networks (RNNs) and their variants, such as Long Short-Term Memory (LSTM), have been employed to create language models that can understand and generate human-like text. This has paved the way for applications like chatbots, virtual assistants, and automated content creation.
Moreover, deep learning has also played a crucial role in the field of drug discovery. By analyzing vast databases of molecular structures and properties, deep neural networks have been trained to predict the efficacy of potential drug candidates. This has accelerated the drug discovery process, reducing costs and the time required for developing new treatments.
Reinforcement Learning and Autonomous Systems
Reinforcement learning has gained tremendous attention in recent years, particularly in the field of autonomous systems. It involves training algorithms to make decisions and take actions based on trial and error, receiving feedback from their environment. This approach has been successfully applied in creating autonomous vehicles, robots, and game-playing agents that can outperform humans in complex tasks.
One of the most notable examples of reinforcement learning is AlphaGo, developed by DeepMind. AlphaGo defeated the world champion Go player, showcasing the power of reinforcement learning in mastering complex strategic games. This achievement demonstrated that algorithms can learn to make optimal decisions by playing against themselves and continuously improving through reinforcement.
Reinforcement learning has also been applied to autonomous vehicles, enabling them to navigate real-world environments and make decisions in real-time. Companies like Tesla have been utilizing reinforcement learning to improve their self-driving capabilities, making vehicles safer and more efficient. By learning from human drivers and the data collected from various sensors, autonomous vehicles can adapt to different road conditions and make intelligent decisions.
In the robotics field, reinforcement learning has allowed robots to learn to perform intricate tasks such as grasping objects, manipulating tools, and even acrobatics. By combining deep learning with reinforcement learning, researchers have developed robotic systems that can learn from scratch and acquire complex motor skills through trial and error.
Transfer Learning and Generalization
Transfer learning has emerged as a powerful technique in the field of machine learning. It enables models to leverage knowledge learned from on e task to improve performance on another related task. This approach has significantly reduced the need for large labeled datasets and accelerated the development of AI applications. Transfer learning has proven to be highly effective in diverse domains, such as computer vision, natural language processing, and speech recognition, where it has been successfully implemented.
In computer vision, transfer learning has enabled the development of pre-trained models that can be fine-tuned for specific tasks. For example, models trained on large-scale image recognition datasets like ImageNet can be adapted for tasks like object detection, image segmentation, and even video analysis. This has democratized computer vision applications, allowing developers to build robust systems with less data and computational resources.
Similarly, in natural language processing, transfer learning has proven to be highly effective. Large-scale language models like OpenAI’s GPT have been pre-trained on vast amounts of text data and can be fine-tuned for specific tasks like text classification, named entity recognition, and sentiment analysis. This has simplified the development of language-based applications, empowering developers to create sophisticated NLP systems without starting from scratch.
Transfer learning has also been applied to speech recognition tasks. By pre-training models on large-scale speech datasets and then fine-tuning them for specific speech recognition tasks, researchers have achieved remarkable accuracy in speech-to-text transcription. This has facilitated the development of voice-controlled systems, transcription services, and even applications for individuals with speech impairments.
Explainability and Ethical AI
As AI and ML technologies become more sophisticated and pervasive, the need for explainability and ethical considerations has become increasingly important. Researchers and practitioners are working on developing techniques that provide transparency and interpretability in AI systems. This ensures that decisions made by AI algorithms can be understood and trusted by humans. Ethical AI frameworks are also being developed to address issues such as fairness, accountability, and bias in AI decision-making processes.
Explainability is particularly crucial in critical domains like healthcare, finance, and autonomous systems. Patients and doctors need to understand the reasoning behind AI-driven medical diagnoses and treatment recommendations. Similarly, users of financial services should be able to comprehend the factors considered by AI algorithms when making loan approvals or investment decisions.
Various approaches have been proposed to improve the explainability of AI systems. This includes techniques like attention mechanisms, which highlight the most important features or inputs that influenced the decision-making process. Researchers are also exploring methods like rule extraction, which aims to generate human-readable rules based on the decisions made by complex AI models.
In terms of ethical considerations, efforts are being made to ensure that AI systems are fair, unbiased, and accountable. This involves auditing datasets to identify and mitigate biases, ensuring diverse representation during model development, and establishing clear guidelines and regulations for the use of AI in sensitive areas like criminal justice and hiring practices. By addressing these ethical challenges, AI can be harnessed to benefit society while minimizing potential harm.
Edge Computing and Federated Learning
Edge computing, coupled with federated learning, has emerged as a promising approach to address the challenges of processing and analyzing vast amounts of data in real-time. Edge computing brings computation closer to the data source, reducing latency and enhancing privacy and security. Federated learning allows multiple edge devices to collaboratively train machine learning models without sharing raw data. This approach is particularly beneficial in scenarios where data privacy is a concern, such as healthcare and finance.
Traditionally, data processing and analysis have been centralized, requiring data to be transmitted to remote servers for analysis. However, this approach can lead to latency issues, privacy concerns, and increased network bandwidth usage. Edge computing solves these challenges by performing computations at the edge of the network, closer to where the data is generated.
Federated learning takes the concept of edge computing further by enabling collaborative model training across multiple edge devices. Instead of sending raw data to a central server, the models are trained locally on the edge devices, and only the model updates are aggregated. This approach ensures that sensitive data remains on the edge devices, addressing privacy concerns while still benefiting from the collective intelligence of the network.
The combination of edge computing and federated learning has significant implications for healthcare applications. For example, wearable devices can continuously monitor a patient’s vital signs and perform real-time analysis to detect anomalies or potential health risks. By keeping the data on the device and leveraging federated learning, privacy is maintained while allowing the development of accurate predictive models for personalized healthcare.
In conclusion, the advancements in AI and ML are shaping the future in numerous ways. Deep learning, reinforcement learning, transfer learning, explainability, and edge computing are just a few of the exciting areas where significant progress has been made. As these technologies continue to evolve, it is crucial to prioritize ethical considerations and ensure that AI systems are transparent, interpretable, and unbiased. With the rapid pace of innovation, we can expect AI and ML to have an even more profound impact on our lives, unlocking new possibilities and transforming industries in the years to come.